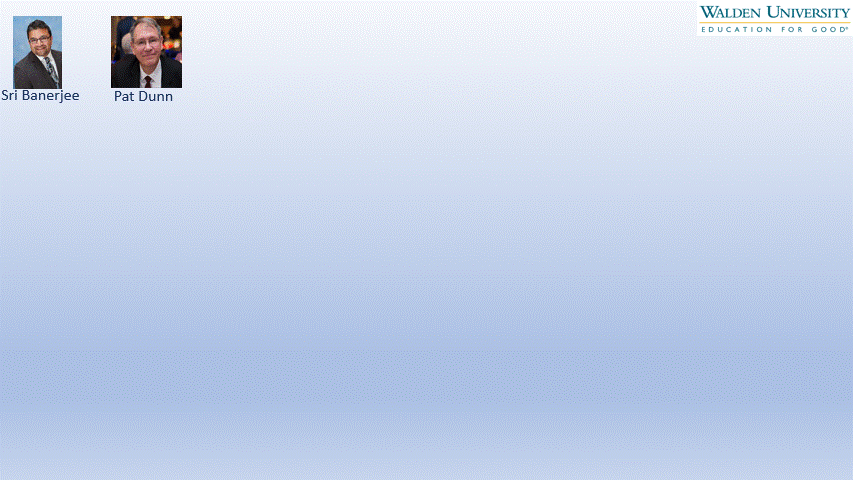
The figure above represents the order to relative importance of the risk factors. For instance, lack of employment and depression play a role in risk prediction of hypertension. These are lambda optimized models and a result of ensemble AI models. We are currently actively pursuing applications of AI that are in the fields of public health, education, and healthcare. Understanding the importance of disease factors in risk prediction can allow for better prioritization which conditions are assessed by healthcare providers and which issues can be addressed in which order. Please see the abstract that was published in this link and video of the full presentation that was delivered at the national Conference at Statistical Practice.
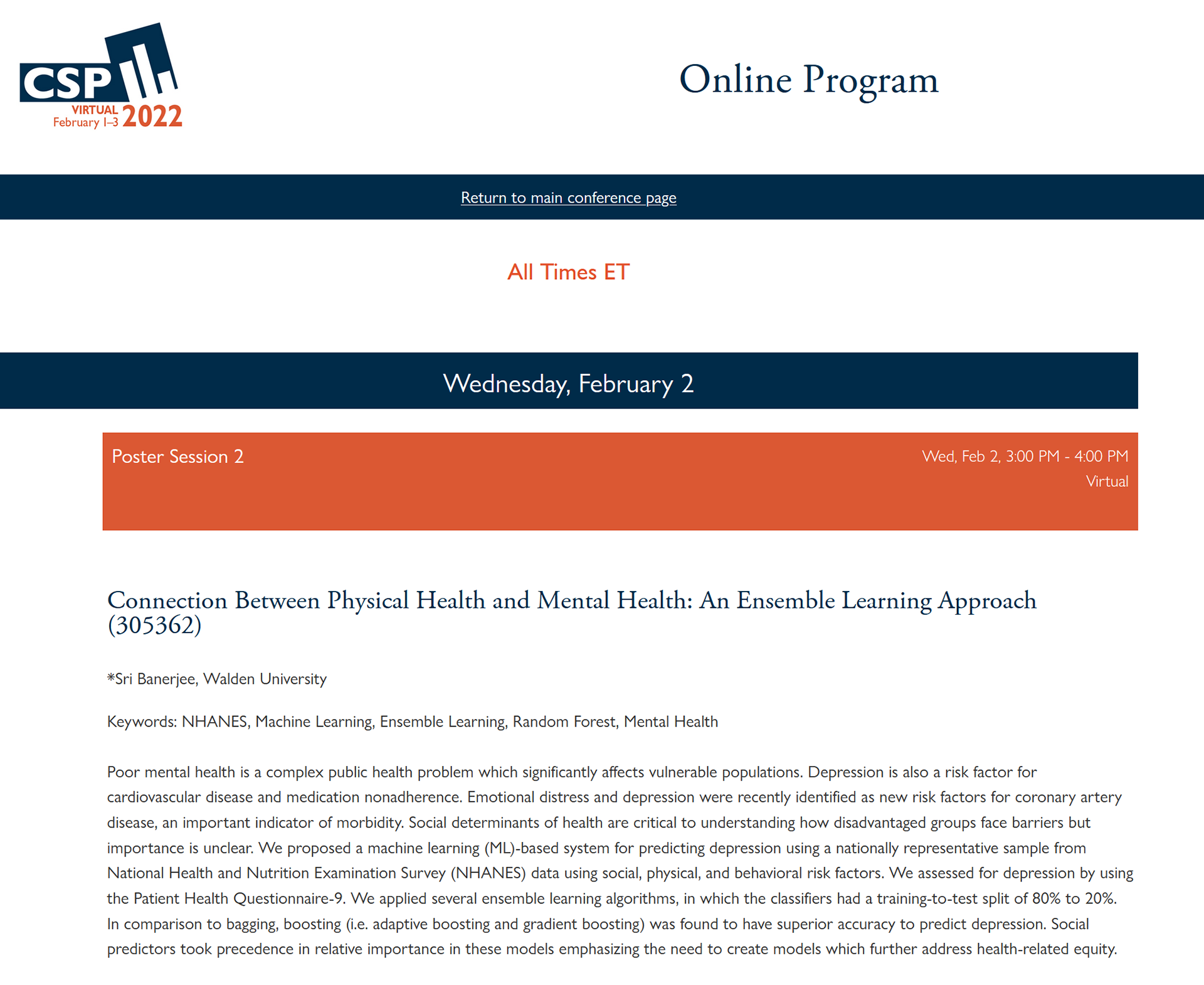
Above is a technique mentioned on how to use artificial intelligence to guide social media analysis. There are several qualitative themes that can be gathered from the social media. I discuss with director of IRB Drs. Leilani Gjellstadt and Lee Stadtlander providing details.
Special Message for Artificial Intelligence from the Lab Director:
We are currently involved in leveraging and harnessing the power of Artificial Intelligence (AI) and machine learning in health research. With massive amounts of data flowing from medical records, wearables, and countless smart home devices, the potential for machine learning and AI to transform healthcare is perhaps more drastic and profound than any other industry out there. Imagine the possibilities of better preventing asthma by measuring the instantaneous air quality within the home. However, there are unique obstacles that exist in healthcare and population health that can make it difficult to apply machine learning. Some of these obstacles revealed themselves during the pandemic. Oftentimes, data are missing, inaccurate or stored in silos. Connecting patient records across providers and insurers is a challenge due to the lack of interoperability and reliable patient identification methods.
We have presented several machine learning models at regional and national conferences like the Upstate Statistical Conference and the annual Joint Statistical Meeting at the American Statistical Association.
Natural Language Processing (NLP) is one such application to complete sentiment analysis and other word recognition tools for data mining and qualitative research. In simple terms, NLP enables machines to understand the human language. Its goal is to build systems that can make sense of text and automatically perform tasks like translation, spell check, or topic classification.
Alternatively, machine learning can be used for classification of specific diseases and precision medicine. Better diagnosis will also lead to better prognosis and also improved prevention which is the eventual goal of public health. This is where AI steps in. We have presented our diabetes random forest risk prediction model. We plan to use other supervised machine learning techniques to better understand the connection with health.
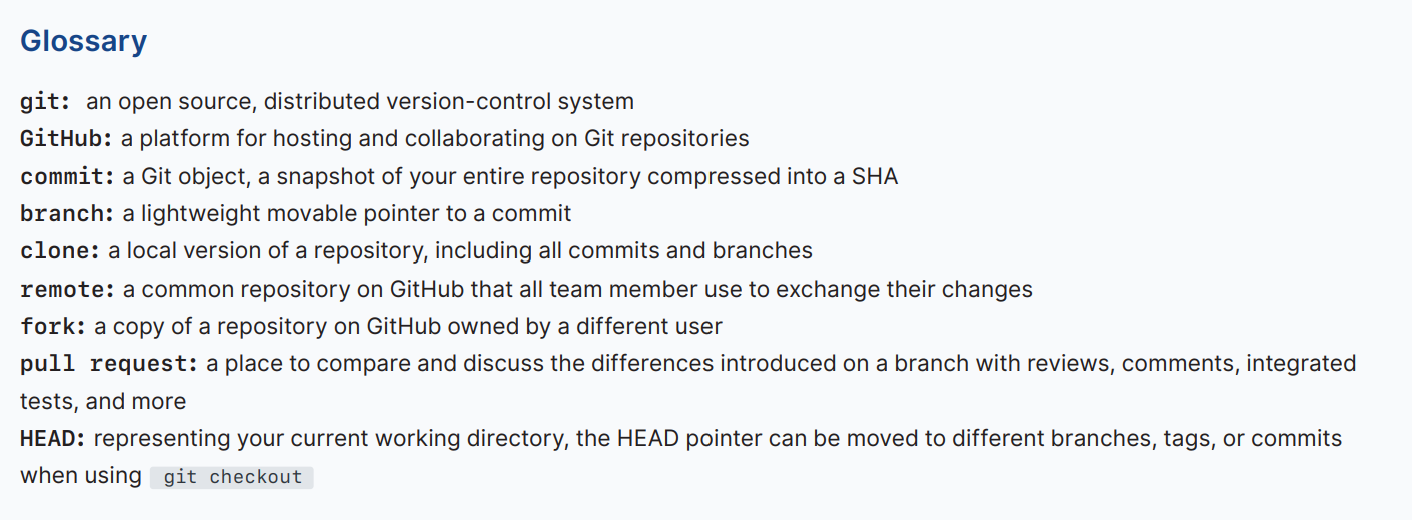
I recently saw a certificate program in Business Intelligence and thought that these would be great topics for potential for curriculum:
Big Data
Data Mining
Supervised Learning
Unsupervised Learning
Predictive Analytics
Reinforcement Learning
Deep Learning
Natural Language Processing